You’re about to embark on a journey into the world of patient data analysis. In this comprehensive guide, we’ll take you step-by-step through the process of analyzing patient data, equipping you with the skills and knowledge necessary to delve into this invaluable resource. Whether you’re a healthcare professional, researcher, or simply curious about the power of data, this guide is designed to demystify patient data analysis and help you unlock its potential. So, grab your notepad and get ready to dive into the exciting world of analyzing patient data.
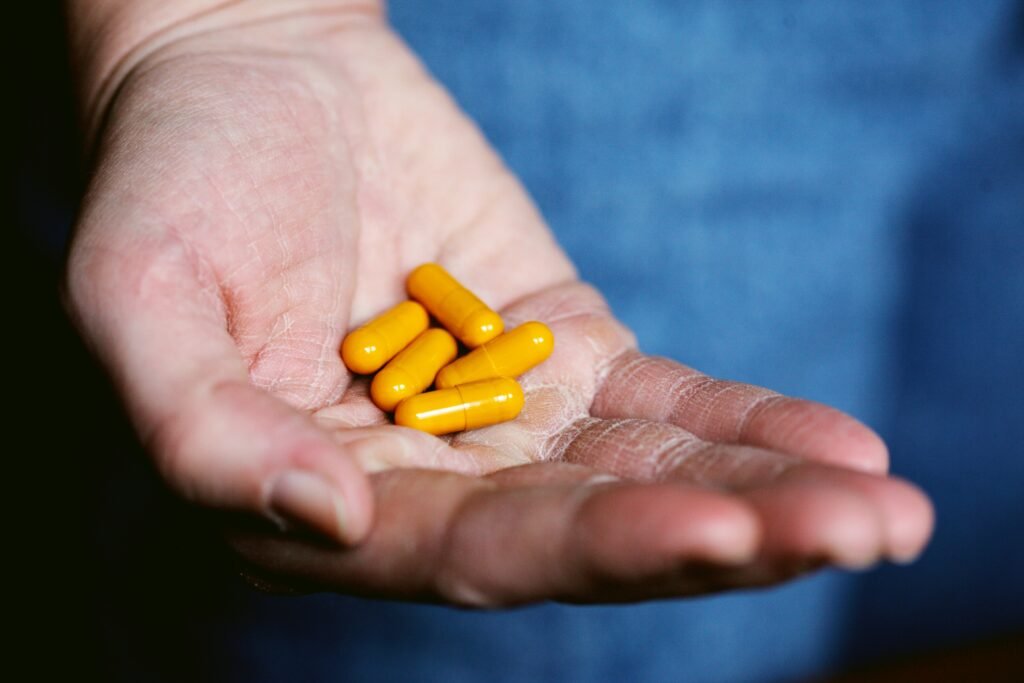
This image is property of images.unsplash.com.
Check out our Product Reviews
A Comprehensive Guide to Step-by-Step Patient Data Analysis
Patient data analysis plays a crucial role in healthcare, helping medical professionals gain valuable insights and make informed decisions. In this comprehensive guide, we will take you through the step-by-step process of analyzing patient data, from collecting the data to presenting the analysis findings. So let’s dive in!
1. Collecting Patient Data
1.1 Types of Patient Data
Collecting patient data is the first and most crucial step in the analysis process. Patient data can be categorized into several types, including demographic data, clinical data, diagnostic data, and treatment data. Demographic data includes information such as age, gender, and ethnicity, while clinical data covers medical history, symptoms, and diagnoses. Diagnostic data involves lab test results and imaging reports, while treatment data includes medications, procedures, and outcomes. By collecting these diverse types of data, healthcare professionals can gain a comprehensive view of each patient’s health.
1.2 Methods of Data Collection
There are various methods to collect patient data, and the choice of method depends on several factors such as the size of the healthcare facility and the specific requirements of the analysis. Some common methods include electronic health records (EHRs), patient surveys, medical devices, and wearable sensors. EHRs provide a centralized repository of patient information, making it easily accessible for analysis. Patient surveys can gather subjective information directly from the patients themselves. Medical devices and wearable sensors can capture real-time data, enabling continuous monitoring of patients. By utilizing these methods, healthcare professionals can collect a vast amount of data for analysis.
Check out our Product Reviews
2. Preparing Patient Data for Analysis
Before diving into the actual analysis, it is crucial to prepare the patient data to ensure its accuracy and quality.
2.1 Data Cleaning and Validation
Data cleaning involves identifying and correcting any errors or inconsistencies in the data. This process may include removing duplicate entries, fixing missing values, and resolving data entry errors. Validation ensures that the collected data meets certain criteria and standards. It involves checking data accuracy, completeness, and consistency.
2.2 Data Transformation
Data transformation refers to the process of converting raw data into a format suitable for analysis. This may involve normalizing data, standardizing data units, and encoding categorical variables. By transforming the data, healthcare professionals can ensure that it is consistent and ready for analysis.
2.3 Data Integration
In some cases, data from multiple sources may need to be merged or integrated to create a comprehensive dataset. This allows for a more holistic analysis, providing a broader understanding of the patient population. Data integration requires careful consideration of data compatibility and the establishment of appropriate data linkage methods.
3. Choosing the Right Analysis Method
Once the patient data is prepared, it’s time to choose the appropriate analysis method based on the objectives of the analysis and the available data.
3.1 Descriptive Analysis
Descriptive analysis involves summarizing and describing the collected data. It provides an overview of the patient population, including characteristics and trends. Descriptive analysis methods include calculating measures of central tendency, such as mean, median, and mode, as well as measures of dispersion, such as variance and standard deviation.
3.2 Inferential Analysis
Inferential analysis aims to draw conclusions about a larger population based on a sample of data. It involves hypothesis testing, where statistical tests are used to determine the likelihood of a hypothesis being true. Confidence intervals help estimate the precision of inferential analysis results. Regression analysis is also commonly used in inferential analysis to explore relationships between variables.
3.3 Predictive Analysis
Predictive analysis utilizes statistical and machine learning techniques to make predictions or forecasts about future events or behaviors. It involves training models on historical patient data and using these models to predict patient outcomes or behaviors. Common predictive analysis techniques include machine learning algorithms, time series analysis, and forecasting models.
3.4 Prescriptive Analysis
Prescriptive analysis goes beyond predicting future outcomes and provides recommendations on the best course of action. It utilizes optimization techniques, decision trees, and simulation models to identify the most optimal decision or treatment plan for individual patients or the overall patient population. Prescriptive analysis helps healthcare professionals make data-driven decisions and optimize patient care.
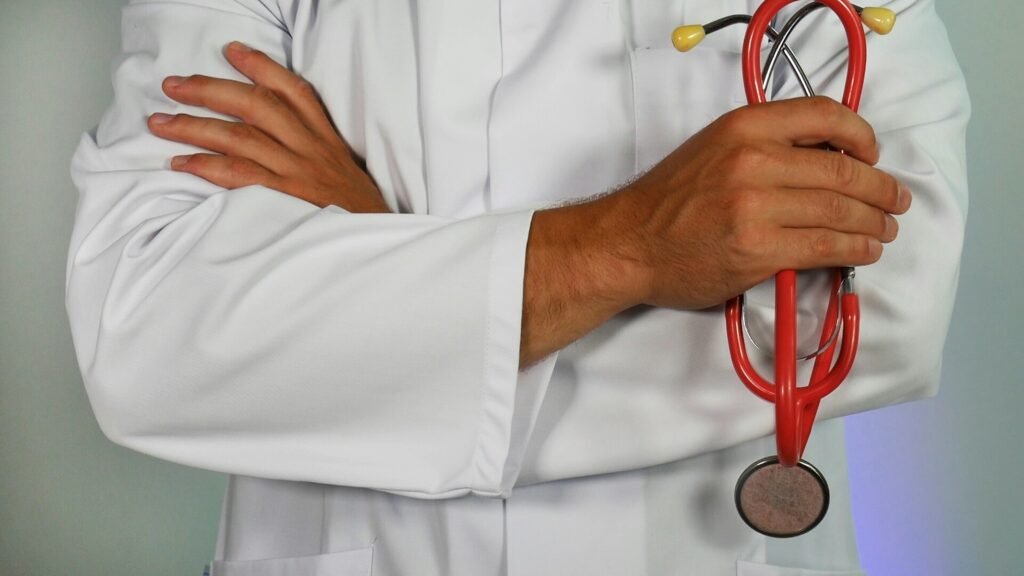
This image is property of images.unsplash.com.
4. Conducting Descriptive Analysis
Descriptive analysis provides insights into the patient population and their characteristics.
4.1 Measures of Central Tendency
Measures of central tendency, such as the mean, median, and mode, help summarize the central or average value of a set of patient data. The mean represents the arithmetic average, while the median is the middle value of a sorted dataset. The mode refers to the most frequently occurring value. These measures provide a quick overview of the data distribution.
4.2 Measures of Dispersion
Measures of dispersion, such as variance and standard deviation, quantify the spread or variability of the patient data. Variance measures the average deviation from the mean, while the standard deviation indicates the extent to which data points deviate from the mean value. These measures enable healthcare professionals to understand the variability within the patient population.
4.3 Data Visualization
Data visualization is a powerful technique to present patient data in a visual format. Charts, graphs, and other visual representations make it easier to interpret complex data and identify patterns or trends. Common data visualization techniques include bar charts, line charts, scatter plots, and heatmaps. Utilizing these techniques, healthcare professionals can communicate their findings effectively and engage stakeholders in the analysis process.
By conducting descriptive analysis, healthcare professionals gain valuable insights into the patient population and their characteristics.
5. Performing Inferential Analysis
Inferential analysis takes the analysis a step further by drawing conclusions about a larger population based on a sample of data.
5.1 Hypothesis Testing
Hypothesis testing involves formulating a hypothesis about the patient population and using statistical tests to evaluate the likelihood of the hypothesis being true. It helps determine if the observed differences between groups are statistically significant or simply due to chance. Common hypothesis testing techniques include the t-test, chi-square test, and ANOVA.
5.2 Confidence Intervals
Confidence intervals provide a range of values within which the true population parameter is likely to fall. They help estimate the precision and reliability of inferential analysis results. A narrower confidence interval indicates higher precision, while a wider interval suggests higher uncertainty. Confidence intervals are essential in providing a range of plausible values for any statistical inference.
5.3 Regression Analysis
Regression analysis is used to explore relationships between variables and predict outcomes. It helps identify how changes in one variable affect another, enabling healthcare professionals to understand the impact of different factors on patient outcomes. Regression analysis techniques range from simple linear regression to more complex models such as logistic regression and multiple regression.
By performing inferential analysis, healthcare professionals can make meaningful conclusions about the patient population based on a smaller sample of data.

This image is property of images.unsplash.com.
6. Utilizing Predictive Analysis
Predictive analysis takes advantage of statistical and machine learning techniques to make predictions or forecasts about future patient outcomes or behaviors.
6.1 Machine Learning Algorithms
Machine learning algorithms, such as decision trees, random forests, and neural networks, can be trained on historical patient data to predict outcomes or behaviors. These algorithms learn patterns in the data and use them to make predictions on new, unseen data. Machine learning enables healthcare professionals to identify patients at risk, personalize treatment plans, and improve patient outcomes.
6.2 Time Series Analysis
Time series analysis is used when patient data is collected at regular intervals over time. It helps identify patterns and trends in the data and forecast future values. Time series analysis techniques include autoregressive integrated moving average (ARIMA) models, exponential smoothing, and Fourier analysis. By utilizing time series analysis, healthcare professionals can anticipate patient needs and optimize resource allocation.
6.3 Forecasting Models
Forecasting models are used to predict future patient outcomes or events based on historical data. These models take into account various factors and utilize statistical techniques to estimate the likelihood and timing of future events with a certain degree of accuracy. Forecasting models can be particularly useful in predicting disease outbreaks, patient readmissions, and healthcare resource demands.
By utilizing predictive analysis, healthcare professionals can proactively address patient needs, optimize treatment plans, and improve overall healthcare outcomes.
7. Implementing Prescriptive Analysis
Prescriptive analysis provides recommendations on the best course of action based on the analysis results.
7.1 Decision Trees
Decision trees are a popular prescriptive analysis technique that helps healthcare professionals make optimal decisions. Decision trees create a visual representation of possible decision paths and their outcomes. By evaluating different decision options and their probabilities, healthcare professionals can identify the most effective treatment plans or interventions for individual patients.
7.2 Optimization Techniques
Optimization techniques aim to find the best possible solution to a given problem, considering constraints and objectives. These techniques can be applied to resource allocation, scheduling, and treatment planning. By optimizing resource allocation, healthcare professionals can maximize efficiency and ensure that patients receive the right care at the right time.
7.3 Simulation Models
Simulation models replicate real-world scenarios and allow healthcare professionals to evaluate the impact of different decisions or interventions. These models take into account various factors and simulate their effects on patient outcomes or system performance. By utilizing simulation models, healthcare professionals can explore different “what-if” scenarios and make informed decisions to improve overall healthcare delivery.
By implementing prescriptive analysis, healthcare professionals can optimize patient care, improve resource allocation, and make data-driven decisions.
8. Interpreting Analysis Results
Interpreting analysis results is a crucial step in the patient data analysis process. It involves drawing insights and identifying patterns or trends.
8.1 Drawing Insights
Drawing insights from the analysis results involves extracting meaningful information and understanding its implications. By analyzing patient data, healthcare professionals may uncover risk factors, potential treatments, or opportunities for intervention. These insights help guide decision-making and drive continuous improvement in patient care.
8.2 Identifying Patterns and Trends
Identifying patterns and trends in the patient data is essential for understanding the underlying dynamics and making informed decisions. Through analysis, healthcare professionals may identify seasonal patterns, demographic trends, or correlations between different variables. These patterns and trends provide valuable insights into the patient population and can inform future strategies and interventions.
By interpreting analysis results, healthcare professionals gain a deeper understanding of the patient population and its dynamics.
9. Presenting Analysis Findings
Presenting analysis findings effectively is crucial for communicating insights and facilitating decision-making.
9.1 Data Visualization Techniques
Data visualization techniques help present complex analysis findings in a visually appealing and easy-to-understand format. Bar charts, line charts, heatmaps, and scatter plots are just a few examples of visualization techniques that can effectively convey information to stakeholders. By utilizing data visualization techniques, healthcare professionals can engage stakeholders and effectively communicate the key findings of the analysis.
9.2 Creating Reports and Dashboards
Reports and dashboards provide concise summaries of the analysis findings, making it easy for stakeholders to access and interpret the results. Reports can include executive summaries, key findings, and detailed analysis results. Dashboards, on the other hand, provide an interactive interface that allows stakeholders to explore the data and insights in real-time. By creating reports and dashboards, healthcare professionals ensure that the analysis findings are accessible and actionable.
By presenting analysis findings effectively, healthcare professionals can facilitate decision-making and drive improvements in patient care.
10. Ensuring Data Privacy and Security
Patient data privacy and security are of utmost importance throughout the entire data analysis process.
10.1 Anonymization Techniques
Anonymization techniques are used to remove personally identifiable information from the patient data, ensuring patient privacy. Techniques such as de-identification and pseudonymization help protect patient identities while maintaining the usefulness of the data for analysis. By anonymizing patient data, healthcare professionals can comply with privacy regulations and safeguards while still gaining valuable insights.
10.2 Encryption Methods
Encryption methods are used to protect patient data from unauthorized access during storage or transmission. Encryption algorithms ensure that only authorized individuals can access the data and that it remains secure even if it falls into the wrong hands. By encrypting patient data, healthcare professionals can maintain data confidentiality and protect patient privacy.
10.3 Compliance with HIPAA Regulations
Compliance with HIPAA regulations is essential for ensuring the privacy and security of patient data. The Health Insurance Portability and Accountability Act (HIPAA) sets standards for the protection of sensitive patient information and establishes guidelines for data handling. Adhering to HIPAA regulations helps healthcare professionals maintain patient trust and prevent potential legal and reputational consequences.
By ensuring data privacy and security, healthcare professionals can protect patient information and maintain trust in the analysis process.
In conclusion, patient data analysis is a multifaceted process that involves collecting, preparing, and analyzing data to gain valuable insights into the patient population. By following the step-by-step approach outlined in this guide, healthcare professionals can make data-driven decisions, optimize patient care, and drive continuous improvement in the healthcare system. Remember to ensure data privacy and security at every stage of the analysis process, complying with regulations such as HIPAA. So start harnessing the power of patient data analysis today and unlock the potential for better healthcare outcomes.
Check out our Product Reviews